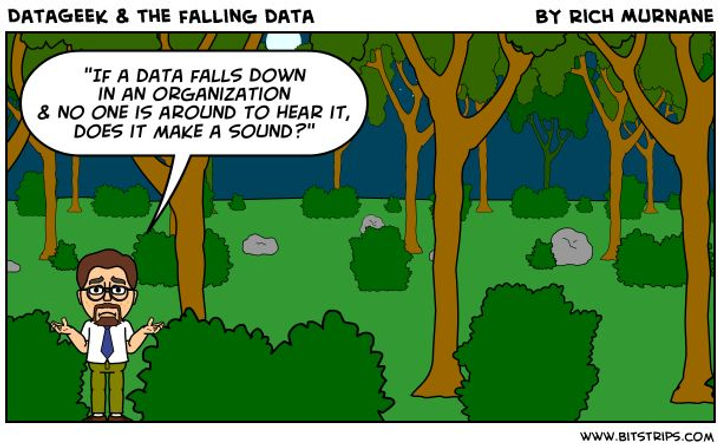
Trusted Data, It’s Elementary
Since March 2020, it seems as if everyone has become aware of the importance of data-driven decisions, as the metrics of COVID-19 became a part of every conversation. Even my mom could articulate the importance of ‘positive tests rate’ and why it is a more significant metric than ‘daily new cases’.Health organizations around the world shared the pandemic data with the public. This data democratization policy was supposed to create transparency and make the decisions trustworthy. However, it was a massive data operation, and as such, it suffered from data reliability issues. The most memorable one was the spreadsheet limit data loss in the UK. But, in my Twitter feed people who were analyzing the Israeli MOH data found mistakes on a weekly basis, and I assume it happened everywhere.At the time, I thought the media coverage of these incidents was exaggerated. Most of these issues didn’t have a significant impact on the metrics and on decision making. Furthermore, it is reasonable to have some mistakes here and there, even embarrassing ones, in such challenging conditions. But in retrospect, the impact of these issues was much more significant than I thought at first, and after years of working with data, it made me think about the true cost of data reliability issues.
“This can’t be right”
I was passionate about data before I even knew what it was. When I was in 8th grade, I was obsessed with playing ‘Football Manager’, and I meticulously maintained spreadsheets with players’ metrics and statistics. My decision making process on how much time to spend on gaining fictional championships could have been better, but the decisions I had made in the game itself were completely data-driven. Despite that, I kept feeling that there was something wrong with the data, often results didn’t make sense.Years later, I learned that ‘Football manager’ has “hidden metrics”, which are not exposed to gamers and affect results. I can unwillingly admit that this made the game entertaining, but in the countless times in my professional life when I felt data didn’t make sense, there was nothing entertaining about it. Every data consumer experienced the frustrating moment when you look at the numbers and think “this can’t be right”.
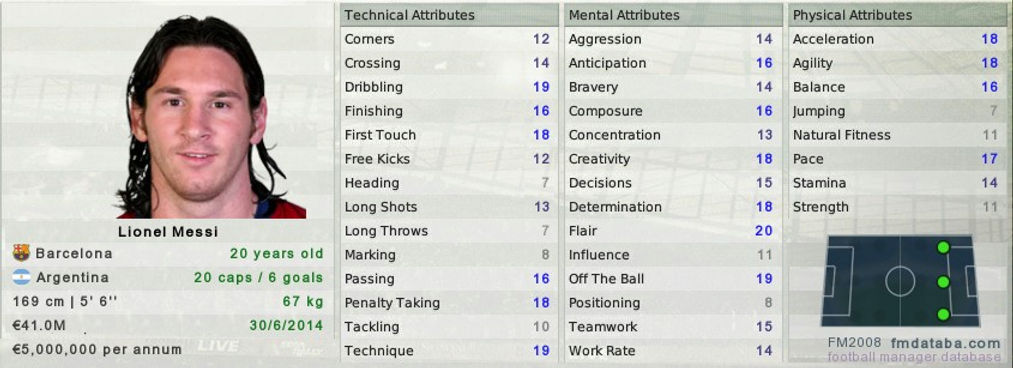
Data breaks
There aren’t intentional “hidden metrics” in real life data stacks, but there are many underlying factors affecting data reliability. Even in relatively small organizations, working with data requires numerous technologies, and processing data from different sources, formats, and types. It requires orchestrating many phases of processing (it’s called pipelines for a reason), and involves professionals with diverse expertise and skills.A dynamic and complex data stack is powerful. It enables to quickly adapt to the requirements of organizations that are becoming more and more sophisticated in their use of data.A dynamic and complex data stack is also prone to errors — Issues that go under the radar and have a cascading effect, changes that have an unexpected impact, human errors, and the list goes on. We came to accept such problems as an inherent part of any data stack, especially as most of them are “noise”, not really significant in the grand scheme of things. It’s hard to improve data reliability, prevent incidents, detect on time, and recover fast. It often feels like it is not worth the effort.The “fake news” of data stacksAs data people, we tend to stick to numbers and measure the severity of problems in our data based on impact. But by measuring it only by the immediate results of an incident, we forget the reason we rely on data in the first place. We consider data to be the most accurate representation of reality, not subject to opinions or biases.
People trust data to be true.
Even if specific incidents are insignificant, the notion of data being the truth is harmed. The trust is breached, and data consumers develop doubts. The COVID-19 data incidents led people to doubt the reliability of every metric that was shared with the public. If these are the mistakes we found, what is being missed? Can we trust any of it?Similar things happened in the pandemic with fake news. The relatively low rate of false stories that made it to mainstream media made the authenticity of every story questionable.
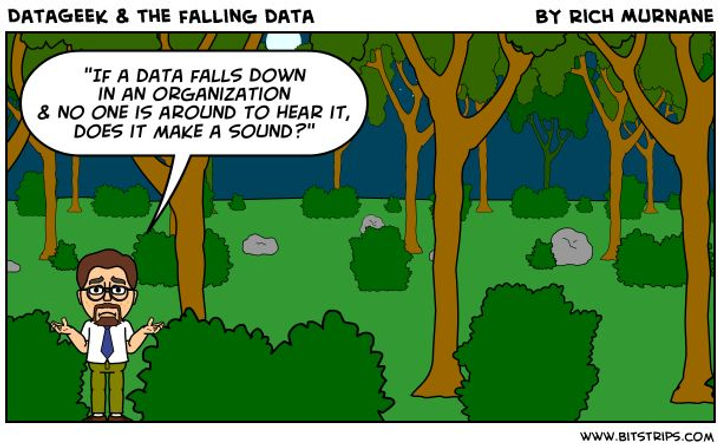
If you have a data stack (in the forest) that consumers don’t trust, is it worth having?
Trust is easy to lose and hard to regain. Data teams that will effectively fight and minimize the “fake news” they deliver to their data consumers will make their entire work more valuable.